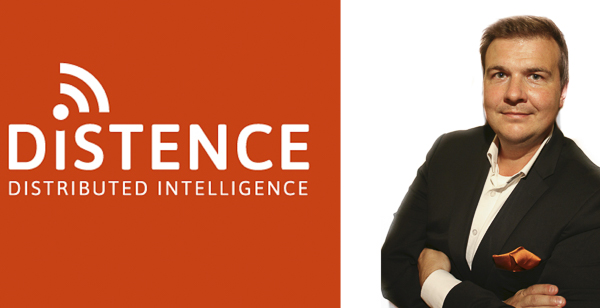
Considering the importance to any production process, the complexity of almost any industrial operation and the effectiveness of proactive maintenance is hard make short and simple if you can exclude full production stoppage from your calculations. It is obvious that significant impact comes from avoidance of downtime, yet this is only part of the total value.
Understanding the relevance and impact to perform right operations at optimal time on a larger fleet of different industrial machinery requires a thorough look into both direct and indirect costs, the IT-infrastructure, the maintenance processes and above all the strategy that guides all the above. It is for this reason that senior management should take a careful look into the preventive maintenance effectiveness and value they deliver “when nothing happens”.
Some principle questions to prepare:
- What is the impact effectiveness of the preventive team – how do we argue the value that was delivered by actions done at the optimal point?
- Are we ahead of events or scrambling when the alert comes?
- How do we make preventive maintenance decisions? How to make sure it’s not too early “just in case” repair?
- Is my required service and maintenance planning horizon hours, days or months?
- Where does my maintenance operation stand on utilising digital tools to automatise repetitive tasks like inspection routines?
After considering the answers to the above questions, the next question is: What costs do the decisions or operating models carry. What indirect costs are hidden in the way we operate?
Are we following up and arguing how good work our preventive teams and technologies are delivering? – Do we have a standard practise to do aftermath after a repair to evaluate:
Sum of actual repair cost VS potential repair cost
- Production time/device stoppage
- Spare parts + repair services
- Maintenance work + overtime
On top of the delta between actual and potential repair cost, there is the efficiency of daily operations. When focusing on rotating machinery, we claim that many preventive maintenance teams consume a lot of resources on manually inspecting healthy machines (wasted resources) and in parallel find themselves on the situation of colliding priorities due to repairs, measurements and/or other scheduled tasks. This is mainly due we only observe the tip of the iceberg as the preventive process was designed.
The Iceberg model is a great starting point to introduce the depth of the topic. Depending on the size of the operation, the costs get multiplied as the complexity and the sheer volume of transactions increases. Let’s use a concrete example. In the life cycle cost of a pump, the initial cost of the pump represents some 10%-15% of the LCC. The rest include spare parts, maintenance costs and energy – if, we look at the direct costs only. But what lies below the surface? That depends on the maintenance strategy. In a simple exercise, anyone can run through their own operations, ice chunk by ice chunk, cost driver by cost driver. The results of this exercise will reveal very different results, depending on whether your strategy is reactive or proactive.
Modern SaaS-based tools have several benefits for them. We can pick out a few that walk hand in hand with the Ice Berg Model. One, they run in the cloud + edge of the cloud, significantly lowering the need and the cost of IT infrastructure. They are available everywhere anytime, globally – fast to roll out. And so is the data and respective information – centralised by default – available to all interested stakeholders.
Sophisticated tools like Condence.io that have a wide range of methods to detect early symptoms of potential failures, e.g. frequency range in vibration analysis and a large toolkit of analysis tools and algorithms. These will move the detection of potentially failing components earlier, from days to months, and allow for forecasting and tracking, thus enabling a proactive strategy – again globally from one User Interface, moving the detection point earlier and automatic. The ability to track specific parameters translates to time – time for planning. This will help preventive teams to focus on machines and conditions that require attention and plan when to take actions.
Planning results in better resource allocation and cost management as well as budgeting and transparency for the future. Transparency to budding issues, the early symptoms of potential problems results in both less downtime, surprises and increases safety. Failing assets also consume more energy as the performance is not optimal. On top of this, it’s also possible that the root cause of failure creates collateral failures leading to reduced asset life or a replacement. It is generally noted that even only an 8% to 10% increase in temperature over normal working conditions reduces the useful life of an electric motor by half.
Depending on the asset and the nature of the operation, various components of the model apply or carry more weight. An unmanned asset, say a pumping station, is very different from a fully staffed manufacturing facility. They both carry the same issues, just weighted differently. Modern SaaS-based tools work, thanks to their extreme flexibility, in both.
Are you interested to hear more? – Follow up on Linkedin or get in touch with the @distence team. I’d also like to hear your comment and opinion.
References & further readings:
The Computerized Maintenance Management System, An Essential Tool for World Class Maintenance – Michael Wienker, Ken Henderson, Jacques Volkerts